Insurers have long wrestled with the question of whether it is better for them to buy or build their information technology; that is, whether it is better for them to develop their own proprietary in-house automation or utilize systems developed by outside vendors, with modifications.
Executive Summary
With the abundance of data and analytical resources, how important is it for insurance carriers to develop and maintain those resources in-house? To what extent can carriers effectively rely on third-party providers? To explore this topic of buy vs. build in carrier data analytics, Carrier Management submitted a series of questions to three individuals—one with a major insurance carrier, another with a major reinsurer and the third with a leading actuarial firm—each a key player in helping carriers make strategic decisions about the selection and utilization of data analytics.In the age of big data, the question of “buy vs. build” has moved into the realm of data analytics. Where data was once relatively scarce and difficult to gather, the world is now flooded with private and public data, along with previously unimaginable power to organize and analyze that data.
In light of the abundance of data and analytical resources, how important is it for insurance carriers to develop and maintain those resources in-house? To what extent can carriers effectively rely on third-party providers?
The answer will differ from carrier to carrier, of course, and probably among product lines within a carrier. But the question is central at a time when competition is intense for even marginal advantages in pricing. A slight overinvestment here, or an underpriced exposure there, can mean the difference between success and failure.
To explore this topic of buy vs. build in carrier data analytics, Carrier Management submitted a series of questions to three individuals—one with a major insurance carrier, another with a major reinsurer and the third with a leading actuarial firm—each a key player in helping carriers make strategic decisions about the selection and utilization of data analytics.
Q: In the age of big data, how valuable is traditional insurance premium and loss data? To what extent can new forms of structured and unstructured data be used in its place?
Risa Ryan, Head of Strategy and Analysis, Reinsurance Division, Munich Re America Inc.: Premium and loss data still provide the foundation for our business. As the underpinnings of company strategies, pricing, risk assessment and profitability, these fundamental data elements can’t be replaced, only enhanced.
Beyond premium and loss records, other traditional data elements, such as policy-level detail and application information, are often used for selecting risks, determining profitability and driving growth. These traditional types of insurance data will continue to play a primary role in quantitative and qualitative analyses performed by carriers and reinsurers.
Carriers can enhance their insurance data substantially by incorporating newly available types of structured and unstructured data into sophisticated algorithms for segmenting customers, supporting underwriting decisions and improving claims outcomes.
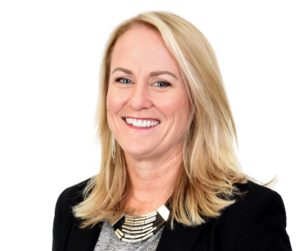
Risa Ryan, Munich Re
Unstructured data, such as documents, reports and email messages related to claims and other client interactions, require text-mining skills and may be more difficult to analyze but share a vast amount of often unrealized potential.
James Korcykoski, Chief Technology and Information Security Officer, Nationwide: We’ve recently been in deliberations over the application of machine learning in underwriting and pricing, and what’s interesting is that the classic data—premium and loss data, the underwriting experience—actually remains just as valuable today as in the past. That data is not easily accessible in the market, and it plays a critical role in our modeling.
However, the relative value of some data elements has changed. Some data we’ve traditionally gathered for writing a new policy and for making underwriting and risk placement decisions is now easy to acquire through alternative data sources.
We also find that external data can be used elsewhere in the value chain. We might handle a specific claim based on something we learn from outside data sources, in addition to the data that we capture at first notice of loss.
Consider, for example, traditional data for commercial lines. We have distinct loss data that tells us how certain types of businesses perform (from a loss perspective), including the causes of loss and their severity. That’s all really valuable data.
Now, a lot of other data—about insured structures, local hazards and more—we can gather from other data sources. We can get that data without having to ask a lot of questions of the buyer, the underwriter or an inspector.
Sheri Scott, Principal & Consulting Actuary, Milliman, San Francisco: I classify data in two categories: the premium and loss data provided by insurers, who are still the best source of it, and other data needed to underwrite and rate a policy that is now available from outside sources.
In fact, it’s rare that you need prior premium or loss data at a very granular level to write a new or renewal policy. What you need is information about the risk you’re writing that helps estimate future losses.
This includes information on the insured property (such as the year a house was built, the year the roof was last replaced, the type of construction and the type of plumbing) plus information on the insureds themselves, such as behaviors that could contribute to potential future loss. Finally, you need information about desired limits and types of coverage.
Most information about a new property or insured have historically been obtained by asking insurance applicants, but buyers are not the best people to provide it. They’re often biased in their responses or don’t know the information accurately.
I’m working with companies that are populating application fields with more reliable data from third-party sources and make for an easier customer experience and provide more accurate data for underwriting, predictive analytics and rating.
Q: What are the biggest advantages to developing and maintaining a storehouse of exclusive, proprietary data? What are the biggest advantages to having in-house data analysts and applications?
Ryan, Munich Re: An organization that develops and maintains its own data will have a competitive advantage over its peers.
Using exclusive or proprietary data to build machine-learning tools allows companies to fine-tune their risk selection and outperform their competitors.
Also, the operational benefits of having in-house analysts and applications can enhance your strategic advantages. In-house analysts work closely with business units and know the data so intimately that they can see connections and develop insights that might not be detected by outside partners.
At Munich Re America, we also use our in-house resources to segment our primary company clients, thereby allowing us to allocate human and capital resources appropriately. Our in-house analysts develop tailored solutions for our clients and position us to bring value to them in areas that were previously untapped.
Having human and technological data resources in-house also allows a company to allocate them flexibly. Analysts can be moved from one project to another in response to internal and external needs far more readily than when relying on external resources.
A strong connection between the analytics team and business stakeholders, and familiarity with company data and systems, makes it easier and more efficient to maintain and update operations and to develop the next generation of systems applications.
Also, the costs of data analysis can usually be better controlled if the data resources are internal.
Korcykoski, Nationwide: We see a lot of value in developing and maintaining a storehouse of algorithms, and those algorithms are informed by a combination of exclusive proprietary data and data available from outside sources.
The fewer questions we ask, the more the customer experience is improved, the more the agent experience is improved. If we can ask fewer questions and still have sufficient data to support our algorithm, that’s a win-win. Some data is worth the extra effort to collect, some data is not, and the art is in knowing which is which.
As for having in-house analysts and applications, think of three ingredients that go into the use of data and analytics for business value: the data itself, the talent to analyze and develop insights from that data, and the technology to analyze and sort the data. Those three elements develop algorithms as their output.
Talent right now is the big challenge. There’s just not enough talent in the market, so it’s critical to acquire that talent and grow it. The technology is readily available. Companies can rent the technology; that’s not something they have to build on their own.
Scott, Milliman: There are definitely advantages to developing and maintaining an in-house data warehouse, including organizing your data optimally for predictive analytics. You can still use cloud services so you don’t have to invest in the hardware, storage and processing needs for big data, and you can have a consulting firm organize and maintain it for you, but you need to maintain ownership of the structure and use of data.
One advantage that new startup insurance companies have is they have a clean slate and can develop well-organized databases for end-to-end needs including analytics. The legacy carriers tend to have old systems and structures, and often their quoting, policy administration and claims systems are separate and not optimally linked.
Having separate systems without an easy way to attach a claim and its details back to the policy and peril providing coverage to a particular insured puts the company at a disadvantage. It is very important for an insurer to set up its own data warehouse with all the appropriate links to various sources of data, including third parties. In doing so, you don’t necessarily have to retain all the data you use in-house, but you have to decide whether you want to or not and optimally design the warehouse.
For example, if you purchased prior loss history on new policyholders and the new property for underwriting, attaching these losses to the policy at the coverage level allows them to be easily used later on for rate development and claims adjusting.
Q: In contrast, what are the benefits and drawbacks of relying on acquired data and outside analytical resources?
Ryan, Munich Re: One size doesn’t fit all companies.
While building a data analytics capability can be the answer for many companies, there is still a case to be made for using external firms to supplement internal capabilities when there is a need to get to the market quickly. In addition, external resources can also offer a fresh perspective on analytic methodologies and an exposure to new and different datasets.
Finally, when analytic capabilities are purchased externally, the vendor commits to your time frame. Developing tools and training users takes time. Often external firms can develop tools and implementation plans including training and execute the plans more quickly than companies can.
A hybrid approach to data analytics is often the right choice.
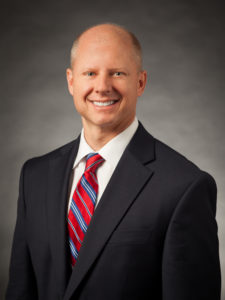
Jim Korcykoski, Nationwide
A burgeoning challenge to management regarding external data is whether it is raw data or derived data. Credit-based insurance scores, for example, are data derived from raw credit reports.
Derived data can get tricky if you utilize risk scores whose calculations change over time but the vendor doesn’t want to disclose proprietary components of the calculation. There’s a risk that an insurer could make decisions that negatively impact its business by relying on derived data it doesn’t fully understand.
For example, there are a lot of companies that will provide data derived from social media postings. You can have a situation where an individual is reported to like guns because an algorithm mistakenly associated the fact that he or she had gone camping with an interest in hunting and guns. If you’re dealing with derived data, you’ve got to be careful about the source.
Raw data is different. There’s nothing that’s massaged or modified on it, so it’s a little easier to trust its value.
Moving on, suppose you decide to have someone outside your company do your data analysis and produce an algorithm. Will it become a commodity? Will the work that went into it be shared with other companies? Will your company ultimately be left without any product differentiation or competitive advantage?
Scott, Milliman: I feel that you need to have both in-house and external data resources.
If you’re not positioned to use third-party data to pre-populate some underwriting and rating fields, I would call you a dinosaur insurance company, and you will lose market share very quickly. You are making the process very onerous for policyholders. Millennials, in particular, don’t want to spend an hour filling out an application and submitting it to be underwritten. For them, it all has to be done immediately and electronically, in real time.
So, I believe that there is a huge benefit to using third-party data sources, and I believe that if you are not doing so now or trying to do so, you will get left behind.
Having said that, there is also a very large benefit to maintaining your own data as well.
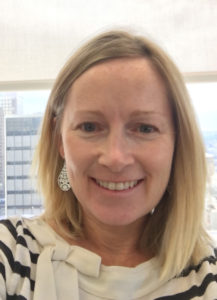
Sheri Scott, Milliman
A new company I’m working with is relying heavily, but not solely, on outside data sources. It has set up an internal data warehouse where it links and tracks all the data used in underwriting and rating. If I want to determine what type of house or what age of house is having a higher or lower closing ratio, or having more or fewer claims, it’s all in their data warehouse.
Using outside analytic resources that have a breadth of experience setting up the processes for various companies can save time and be valuable to companies that don’t have the experience or enough in-house resources.
Q: How much value does your organization place on its proprietary data? Is it a defining feature of your franchise, something you seek for its own sake, or a byproduct of your marketing and underwriting strategy?
Ryan, Munich Re: Our data is our competitive advantage and the key to unlocking future growth and product development for our company and our clients. It factors into how we price our business, how we settle our claims, how we develop new products and even how we acquire new talent. It allows us to deliver the next generation of risk solutions to our clients.
Korcykoski, Nationwide: There is franchise value in having certain data that is unique.
Marketing or sharing data is definitely on a list of the things that we’re thinking about, a value that we have as a company that could be monetized or that we can use to serve our members better.
There are examples of this in the market, and we frequently have conversations with potential business partners about what data we have that might be valuable to them, what data insight they have that might be valuable to us. It’s without a doubt something that we think about.
Scott, Milliman: Insurance companies and agents not only have significant value in their proprietary data but are using their leftover data, or “data exhaust,” as it’s sometimes called.
For example, let’s say you’re running an agency and the insurers you represent don’t want to write in wildfire-prone areas. Rather than just decline those risks, you’ve already collected someone’s name and date of birth, and you’ve probably paid for third-party data to determine if a property is located in a wildfire area. Why not use that to make some money? You could sell that lead to another agent or insurance company that does accept those risks.
Personally, I help companies figure out what data is optimal for them, how to use it and how to integrate it into their operations. Sometimes I even serve somewhat like a data broker, bringing various partners together.
Topics Carriers Profit Loss InsurTech Talent Tech Data Driven Underwriting Reinsurance Training Development