Back when Munich Re launched the industry’s first insurance coverage for artificial intelligence (AI) at the end of 2018, the policy, aiSure, found few takers. In subsequent years, as more companies digitally transformed their business and AI models and machine learning tools went mainstream, business picked up, prompting Munich Re last year to form a dedicated AI insurance team to scale aiSure and develop additional AI insurance policies.
Executive Summary
The global market of AI solutions is poised to skyrocket, and the prospect of having viable risk transfer solutions available to absorb the risks of AI-generated errors may be one more catalyst fueling exponential growth. Munich Re was the first to market with insurance that backs the performance guarantees of AI providers. The coverage serves as an adjunct to the risk identification and mitigation services provided by AI software attestation and audit firms, which are seeing high demand. Insurance Journalist Russ Banham reports on both the services and the novel insurance offerings.Online bonus: Munich Re provides a real-life example of an actual AI risk insured with the aiSure offering.
Why is this important?
Chief among the reasons is that Munich Re’s estimable reputation and clout is sure to inspire other insurers and reinsurers to develop innovative AI-based products, culminating in a new insurance market. Given the rapidly growing use of AI software by companies on a function-by-function basis, viable risk transfer solutions absorbing the risks of AI-generated errors are sorely needed.
The global market for AI solutions is expected to grow from $87 billion in 2021 to $120 billion in 2022, then skyrocket to more than $1.5 trillion by the end of the decade, according to Precedence Research. The market research firm cited rising demand for AI technologies in banking, financial services, manufacturing, advertising, insurance, retail, healthcare, food and beverages, automotive, logistics, robotics, cancer diagnostics, and pharmaceutical. In short, virtually every industry sector.
“All industries are using AI, but they’re still very much at the exponential part of the curve,” said Anand Rao, Global AI lead at Big Four audit and advisory firm PwC. “We’re still somewhat in the early days, with about 20-25 percent of companies deploying AI models to be used by large numbers of people and most of the rest still dabbling.”
Michael Berger, Munich Re
The dabblers will inevitably jump on the bandwagon, hence the importance of Munich Re’s pioneering product. AiSure guarantees the performance of an AI software product. Although the policy covers the technology providers of an AI solution and not the AI users per se, the AI provider can use the insurance backing to offer performance guarantees to its users, essentially de-risking their adoption of AI models.
“Business users of a predictive AI solution to automate their processes or make better decisions might not be able to fully estimate the error probability, resulting in a residual risk,” said Michael Berger, head of the Insure AI team at Munich Re. “We structure a performance guarantee with the AI provider ensuring the model performs to expectations. We then structure a separate insurance policy to contractually transfer their liability over to us, [effectively] de-risking the performance exposures of the AI software to the benefit of users.”
The innovative insurance coverage is designed to alleviate concerns over the reliability of AI model outputs. It also serves as an adjunct to the risk identification and mitigation services provided by AI software attestation and audit firms. Using such services, business buyers of AI products have more assurance of the accuracy and reliability of the tools. An insurance product transferring residual risks is the icing on the cake.
As Martin Eling, a professor of insurance management specializing in the business uses of AI at the University of St. Gallen in Switzerland, put it, “Attestations and audits signal you have reliable technology. Then, if something doesn’t go according to plan, an insurance product would take care of it. That’s good news for users who want to feel they have control over what is going on in this new world of technology.”
Explosive Growth and Risks
Nearly every business today is on a digital transformation journey involving the use of automation, digital, data and analytics solutions. AI models driven by machine learning algorithms are a crucial part of this voyage, as the tools can recognize certain patterns in data, alerting business users to a broad range of risks and opportunities, depending on the type of company and its specific needs. For example, AI models are used to automate and streamline business processes, make product recommendations to customers, autonomously drive automobiles, and robotically assist surgeons.
“All industries are using AI, but they’re still very much at the exponential part of the curve.”
Anand Rao, PwC
That’s the short list. The problem is when the AI predictions or outputs are mistaken. A case in point is an algorithm embedded with unintentionally biased information used in employment decisions. Facial recognition algorithms using old data often tilt primarily toward white men, resulting in lower accuracy rates in correctly identifying the faces of people of color, women and gender-nonconforming people.
Another example is the use of AI to underwrite automobile insurance. Carriers may use old sources of data that include geographic ZIP codes that correlate with a particular demographic, increasing the risk of biased decisions involving people of different ethnic backgrounds. The cognitive biases of algorithm developers also may seep into the models they develop, given that we all have unconscious biases about other people.
Berger from Munich Re provided an example of an erroneous AI model in a manufacturing context. “Many manufacturers’ automated quality control systems depend on visual sensors that analyze a part on a conveyor belt,” he said. “The data from the sensors is interpreted by the AI to spot a defective part.”
Two errors can occur if the AI solution is mistaken. “If the AI says a part is defective but it isn’t, that’s one error. If it says a defective part is not defective, that’s another error,” he said. “The first error results in unnecessary waste and cost; the second can result in defects that require a reputation-damaging product recall and potential product liability.”
Another costly possibility involves merchants in the ecommerce space. If an AI tool designed to detect fraudulent credit card transactions is erroneous, merchants are susceptible to lost income. “If the tool suggests a legitimate transaction is fraudulent, the merchant loses out on business it otherwise would have had,” Berger said.
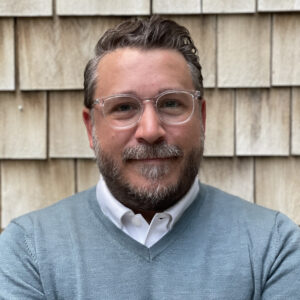
Anthony Habayeb, Monitaur
Last but not least is the use of AI to safely direct self-driving vehicles. Autonomous cars rely on the use of machine learning algorithms to analyze data flowing in from multiple sensors and a variety of external data sources like real-time weather reports, traffic lights, GPS and so on. If the AI solution fails for some reason, a serious accident is possible.
The risk of an “underperforming AI model” is the main reason why nearly three-quarters of companies are still on the fence about the technology, said Rao. “Of the 20-25 percent of companies deploying AI broadly, we’ve noticed that things can potentially go wrong—nothing earth-shattering but concerning nonetheless,” he said, adding that AI insurance is an important step toward greater adoption.
Attesting to the Truth
The risk of AI modeling errors also has ignited the development of AI-focused attestation and audit services firms, Rao said. “In 2016, we began to see a number of tech companies, academics and standards frameworks like NIST [National Institute of Standards and Technology] wrestle with how to verifiably affirm the outcome of an AI model, leading to the increase in this type of work,” he explained.
AI attestation and audit firms provide a range of services to buyers of AI solutions, from assessing, mitigating and assuring the safety, legality and ethics of an AI algorithm to identifying and mitigating bias in AI-generated psychometric and recruitment tools. These services are in high demand, Eling said. “You can’t have an excellent ‘black box’ making excellent decisions without knowing how it came to these decisions. Users want assurance of a linear causal relationship for the outcomes generated.”
This is the type of work conducted by AI governance software provider Monitaur, whose services are used by both attestation and audit firms and insurance carriers. “We were founded with the opinion that enabling governance and compliance as related to machine learning is one of the most ennobling things we could do to realize the absolute good of AI,” said Anthony Habayeb, Monitaur’s co-founder and CEO. “I’m inspired in the belief that machine learning can make everyone’s lives better.”
To seize this good, an attestation or audit firm needs to assure the reliability of the algorithm’s output. “It needs to plot an objectively verifiable audit trail, he explained. “We provide transparency into the decision event—the things the AI model did in making the decision it made.”
In other words, Monitaur peels the onion to its core, one layer at a time, “from the decision event back to the starting point of the software,” Habayeb said. “We have a team here that’s specialized in model assurance, whose job is to organize all this information as a system of record…No one builds perfect software; there will always be a negative event. To manage the risks of AI like other exposures, you need systematic controls.”
Compliance and regulatory factors are other issues intensifying the need for AI risk assessment and quantification. More than 60 nations have adopted some form of AI policy, according to the Brookings Institution, which called it “a torrent of activity that nearly matches the pace of modern AI adoption.”
Regulators in the EU, for example, are in the process of updating European product liability laws for AI providers to assume the risks of damage and bodily injuries generated by their products.
In the U.S., 17 states in 2022 introduced AI bills or resolutions, four of which have been enacted. New York, for example, recently required that employers conduct “bias audits” in AI-generated hiring decisions. Federally, the Biden administration in October 2022 unveiled an AI “Bill of Rights” laying out guidelines for future uses of AI technologies. The government’s goals include limiting the impact of algorithmic bias and ensuring that automated systems are transparent and safe.
Added up, as AI providers up their game to comply with pending rules and future regulations and the many exposures related to more widespread use of the solutions become better understood, quantified and managed, a robust AI insurance market is a likelihood. “Insurance is an exercise in the evaluation of a risk, which in the context of AI is improving,” Habayeb said.
Tomorrow’s AI Insurance Market
At Munich Re, several data scientists abetted by research expertise from Stanford University’s statistics department are deeply engaged in analyzing the predictive performance risks of clients’ AI models.
“Naturally, we’re super excited about working on this project, as it has huge importance to society and businesses,” said Berger. “We’re essentially supporting companies on their digital transformation journey; we’re there to mitigate the risks that arise on this journey, which has only just begun.”
Eling believes that Munich Re itself is at an early juncture in building out its AI insurance solutions. “For Munich Re, the largest reinsurance company in the world, aiSure is just play money,” he said. “They’re not looking to make a big profit; they’re getting in early to understand the critical risks surrounding the growing use of AI. In five or 10 years, they will have accessed valuable data on risk frequency and severity to reliably estimate premiums in what will become a huge market.”
Will other carriers jump into the fray along with Munich Re, effectively seeding the development of a competitive AI insurance marketplace? “Once they are able to put a price tag on AI risks, which will create incentive for more risk-adequate behaviors by AI makers, I’d have to say yes, especially as the best makers rise to the top,” he replied.
Damion Walker, managing director of insurance broker Gallagher’s technology practice, commented that such a market would be helpful to clients down the line. “I’ve not had any inquiries about the need to support AI with insurance, but it would be a great product for the providers,” he said.
“This industry has a long history of helping to promote new products by backing their financial viability,” he added. “In the next 10 years, this will be more than a trillion-dollar industry. A great name like Munich Re supporting these innovations will go a long way toward the acceptance of technologies important to every company.”
As always in business, if there’s a will, there’s a way.
Topics InsurTech Tech Data Driven Artificial Intelligence Market